An inertial navigation system (INS) is the key enabler for stable UAV navigation when the GNSS signal is degraded or unavailable — and that happens a lot. High-rise buildings, dense vegetation, electromagnetic emissions from nearby structures, or targeted UAV jamming frequently cause signal loss and costly equipment loss.
That shouldn’t be your case, however. Recent advances in sensor fusion and artificial intelligence (AI) enable drones to autonomously navigate complex droughts in GPS-compromises and denied environments.
Here are the top four AI navigation solutions for UAVs, available on the market right now.
1. Bavovna
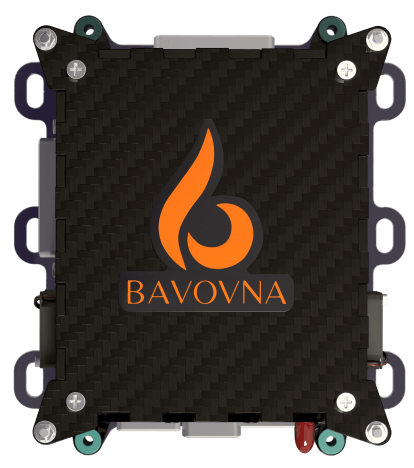
Bavovna offers a versatile AI navigation kit, compatible with multi-rotor, VTOL, and fixed-wing models. The low-SWAP system includes IMU and Al-powered flight control, encased in an EMI-shielded, carbon case (which has been field-tested for reliability). For extra protection, SIGINT RF modules can be added to the INS to identify and overcome EW/EM threats.
The Airtower use case needs to be covered.
Bavovna H-INS got a proven 0.85-meter deviation of single-point positioning without any GPS, RTK, and optical navigation on board, at altitudes 500 meters with a wind up to 18 m/sec.
Unlike other INS, Bavovna’s solution can provide complete autonomous navigation in GNSS-denied environments using a proprietary sensor-fusion AI algorithm. The algorithm is already pre-trained on standard sensor data (multivector aerial speed, multi-vector air flow, aileron feedback, barometer, compass, gyroscope, and magnetometer) to ensure high positional accuracy and situational awareness. Then further Bavovna fine-tune the model, using approximately 100 hours of flight data from a particular airframe to ensure the best possible performance.
Bavovna H-INS stays sensor agnostic and AI-driven sensor fusion can be easily extended to integrate extra navigation aid systems, lidars, or computer vision. For example, if you also want to use simultaneous localization and mapping (SLAM) for mapping the terrain or to use object detection for your applications.
The hybrid approach currently allows Bavovna to provide ultra-low end point positioning error (EPPE) of under 0.5% at a range of 30 km / 18.6 miles — a substantially longer range than non-AI INS and radio-based navigation systems can deliver.
Competitive Strengths
- No GPS, RTK, RC ground, or network signal is required to pilot UAVs using the Bavovna AI navigation system to perform autonomous take-off, Return-To-Home, and landing.
- Sensor fusion algorithm fine-tuned for each customer’s flight gear and application scenarios.
- Modular, scalable unit design with low power consumption, protected with composite radio shielding.
2. George Autopilot
Source: uAvionix
George autopilot system by uAvionix is a robust UAV navigation system, based on certified DAL-C hardware and CubePilot autopilot architecture. Both proved their mettle in a range of enterprise UAV operations.
To power safe flights, George 2 replies on data from the IMU units and military-grade geomagnetic sensors. You also have the option to add detect and avoid (DAA) protection by adding pingRX Pro or ping200X Mode S Transponder. On the downside, George doesn’t use SLAM or odometry to provide extra situational awareness, unlike Bavona.ai.
Another advantage is that the entire navigation system weighs only 80 grams (2.8 oz). It’s also optimized to minimize power consumption for longer flight times and hardened to withstand abnormal power conditions, lightning, and other magnetic interference. The system is certified according to DO-160G and MIL-810H, guaranteeing high resistance to environmental stress.
Competitive Strengths
- Engineered to meet aviation and military environmental standards and compatible with a range of UAVs, including larger VTOL and fixed-wing models.
- George incorporates top-of-time GPS, C2 Radio, IMU, and military-grade sensing technologies, ensuring unprecedented accuracy and situational awareness.
- Plug-and-play solution with an option to integrate additional avionics modules and different ground infrastructure.
3. Spatial FOG Dual
Source: Advanced Navigation
Advanced Navigation is a long-standing leader in inertial navigation systems (INS), offering a range of Micro-Electro-Mechanical Systems (MEMS) and Fiber Optic Gyroscopes (FOG) INS/GNSS solutions. FOGs provide greater accuracy in measuring rotational movements and perform better in dynamic conditions (e.g., rapid maneuvers). They also generate less noisy measurements and require less frequent recalibration.
Spatial FOG Dual combines high-accuracy fiber optic gyroscopes, accelerometers, magnetometers, and a pressure sensor with a dual antenna RTK GNSS receiver. Its EMCORE TAC-450 fiber optic gyro IMU, provides superior inertial data accuracy, surpassing the best MEMS technology. The RTK GNSS receiver provides positioning accuracy of up to 8mm (0.3 in) and a timing accuracy of 20 ns. It also supports post-processing kinematics (PPK).
Similar to Bavovna, Advanced Navigation uses a pre-trained AI neural network sensor fusion algorithm to ensure smooth aerial navigation, even in challenging conditions. The company says its algorithm is 10X more accurate than a traditional Kalman Filter. This allows for extended reckoning, even without GNSS.
The navigation unit is based on a safety-oriented real-time operating system, with all software designed with high fault tolerance in mind. Hardware is rugged and environmentally protected, in line with IP67 and MIL-STD-810G standards.
Competitive Features
- Horizontal position accuracy of 0.01 m with RTK or Kinematica PPK, and velocity accuracy of 0.005 m/s.
- Gyroscopes with a bias instability of 0.1 °/hr and accelerometers with a bias instability of 15 µg.
- Proprietary, pre-trained neural network sensor fusion algorithm that demonstrated strong results in the field.
4. Honeywell Compact Inertial Navigation System
Source: Honeywell
Honeywell Compact Inertial Navigation System (INS) is another great compact option (with a weight of 115 gr/4oz) that packs a punch in terms of hardware. The system includes tactical-grade inertial sensors, dual-antenna GNSS heading, RTK, and closed-loop integration with Pixhawk 2.1 — flexible autopilot. It can be also extended with extra data sources to support specific use cases. For example, you can combine it with a radar velocity system for extra precision or a third-party anti-jamming system for navigating in high EMI environments.
In an RTK configuration, Honeycomb CINS delivers an ultra-low positioning error of 0.03/0.015 meters (and 2. Meters without RKT). The velocity error is just 0.02-0.04 m/s. It’s a great solution for applications where accuracy is critical.
On the downside, Honeycomb doesn’t use AI for sensor data fusion, unlike Bavovna and Advanced Navigation to provide a greater degree of autonomy and reliability in GPS-compromised environments.
Competitive Strengths:
- Compact INS offers high-accuracy output position, orientation, and velocity data, thanks to top-of-the-range hardware.
- Redundant sensors and ruggedized system design lend extra safety to flight missions.
- Easily extendable with extra Honeycomb or third-party navigation aids systems. e.g. velocity aiding systems
TL: DR: Comparison of the Best INS for UAVs
Here’s how the top solutions stack in four key areas: customization, SLAM support, non-GPS navigation, and AI sensor fusion.
Customizable IMU integrations | SLAM support | Non-GPSnavigation | Custom AI sensor fusion algorithm | |
Bavovna AI Navigation Kit | ✔️ | ✔️ | ✔️ | ✔️ |
George Autopilot from Avionics | ✔️ | ❌ | ✔️ | ❌ |
Spatial FOG Dual from Advanced Navigation | ✔️ | ❌ | ✔️ | ✔️ |
Honeywell Compact INS | ✔️ | ❌ | ✔️ | ❌ |